Electric Fleets with On-site Renewable Energy Sources (EFORES)
PI: Dr. Xuewu Dai, Co-I: Richard Kotter, Nauman Aslam, EPSRC-Siemens, 2022-2023,(EP/W028727/1)
This is one of [seven new EPSRC projects that explores how digital technologies and innovation can reduce energy demand and carbon production](https://www.ukri.org/news/studies-to-find-digital-solutions-to-reducing-energy-demand/), sponsored by the Engineering and Physical Sciences Research Council (EPSRC) and Siemens. This project investigates how artificial intelligence and machine learning technologies can provide an optimal dispatching and charging management of electric fleets to improve the efficient use and self-consumption of highly variable onsite renewable energy sources.
Background and Challenges
In line with the UK’s target to reach net zero by 2050, one of the major goals of the EPSRC Energy theme is to increase the efficient use of renewable energy assisted by digital technologies. Electrical vehicles (EVs) charged by renewable energy are one of the solutions towards carbon-neutral road transport, which is the 2nd largest carbon emission both nationally in the UK and locally in Newcastle upon Tyne (contributing about 33% of total CO2e in 2020).
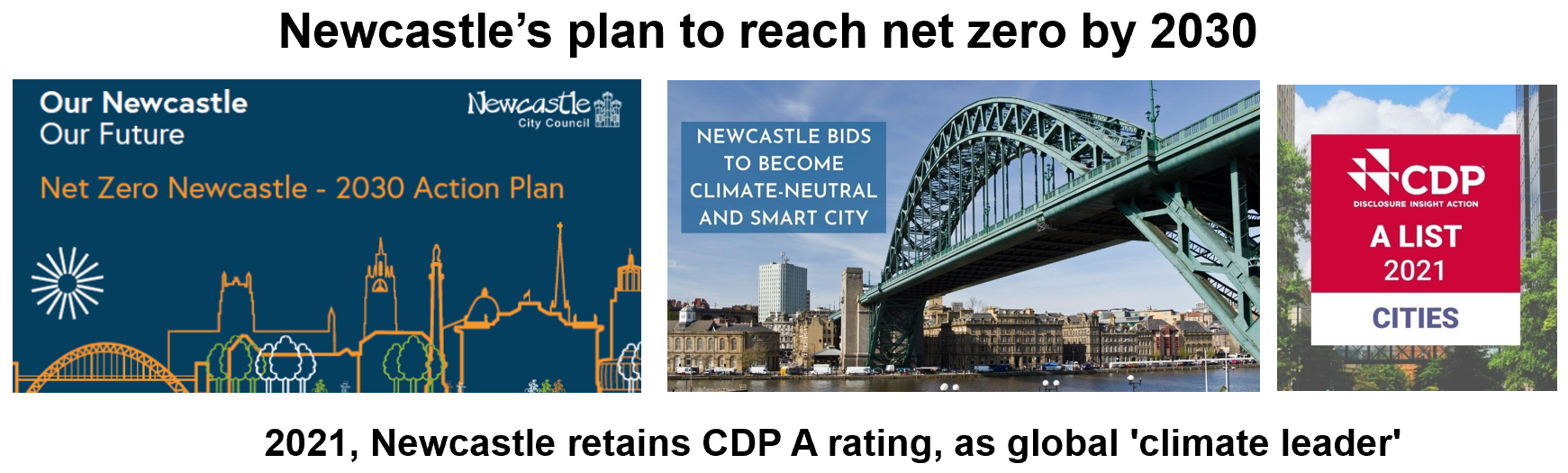
However, more EVs do not automatically imply reduction of overall emission nationally or globally unless their electricity are sourced from renewable energy sources (RES). Despite large-scale major RES projects, a recent trend shows increasing amount of small-scale RES installed on-site, referred to as ORES. For instance, in 2021 Newcastle City Council announced a £27M plan to install solar panels, energy storage etc. at public buildings.This provides opportunities for EV to use more on-site generation renewable electricity to actually reduce the overall emission for road transport. The key issue is the efficient use of ORES.
Aims and Innovation
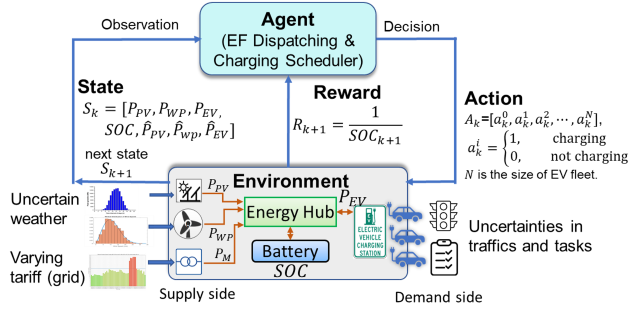
The EFORES project is designed to answer the open question of how to improve the efficient use and self-consumption of highly variable ORES, whilst meeting the dynamic travel demands of collaborative EVs in fleets, at lowest possible investment and operation cost of charging stations and electricity storage facility and least impact on grid peak load. This is addressed by exploring the digital technology of data-driven artificial intelligence and optimization to manage and match the dynamic demands (dispatching and charging of electric fleets) and the supply of ORES - both of which are high variable - to increase ORES utilization and efficiency, thus reducing the actual emission of road transport. The novelty is the development of an empirical data-driven Reinforcement Learning (RL) decision support tool that can provide a near real-time tactical dispatching and charging schedule to improve the self-consumption of ORES.
Project aims are:
- Understanding the spatial-temporal pattern and uncertainties of both EV demand and ORE supply;
- An optimised EV dispatching and charging schedule by developing a RL-based decision making;
- Development of a techno-economic analysis tool to evaluate emission reduction in urban environment.
Research Team
- PI: Dr. Xuewu Dai
- Co-I: Dr. Richard Kotter, Prof. Nauman Aslam
- PDRA: Dr. Handong Li
- Industrial Partners: Scottish Power Energy Networks, Newcastle City Council